Online branding methodology: how Stobbs worked it out
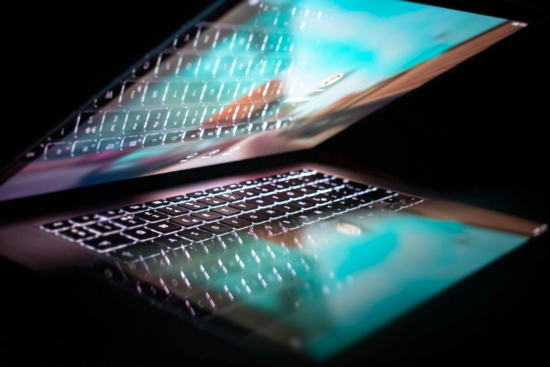
Following the publication of our latest online branding research, we wanted to give readers access to how the calculations were made. So, here is Stobbs’ online branding methodology, here’s how they worked it all out. When it comes to the calculation of online prominence, brand mentions are identified by matching the content of the webpage HTML using ‘regular expressions’ (‘Regex’), a formulation which allows wildcard-based searching and is able to identify brand references within longer strings (such as the URL of the page).
In order to treat all brands identically as far as possible, each classified reference requires the brand name to be preceded and succeeded by a character other than an alphabetical letter (for all brands), to avoid false positives (e.g. where the brand name appears as a sub-string of a longer term). The exception to this point is the inclusion of plurals (e.g. ‘Michelins’ is counted as a mention of ‘Michelin’).
A brand content score is then calculated for each brand on each webpage, based on the total number of mentions and the prominence on the page of each mention. The overall prominence score for each brand is then calculated as the mean of the brand content scores across the set of pages analysed (for those pages which are accessible via the automated analysis script). This methodology has been improved from that used in previous studies in a number of respects, with the most important differences being that the earlier formulation: (i) considered only the absolute numbers of pages on which there was at least one brand mention in each of the key areas of content (URL, title, etc.), without taking account of the number of mentions in each area; and (ii) produced prominence scores which were a function of the total number of pages analysed, requiring the scores to be ‘normalised’ in order to allow comparison between successive studies.
Online sentiment works a little differently. Sentiment is quantified by identifying instances of brand mentions in proximity to any of a library of positive or negative keywords. All keywords are treated equally, with the score-contribution from each brand-reference/keyword pair calculated only as a function of the proximity between the two words, according to an exponentially-decaying function (so that instances where the words appear more closely together will be assigned a higher score), with a ‘positive’ keyword generating a positive score contribution, and vice versa. The total positive and negative scores for each brand can then be calculated, and the overall sentiment score for the brand on the page in question is the difference between the two (i.e. overall sentiment score = positive sentiment score – negative sentiment score).
The analysts then calculated the cube-root of the raw sentiment scores for each brand on each page (to reduce the impact of outliers) and then calculate the mean across all pages on which a reference to that brand was identified. The final sentiment score for each brand is then taken to be this mean value multiplied by the square root of the number of contributing webpages (to provide a measure of significance, and upweighting the score for brands where the mentions are consistently positive or negative, and downweighting it for brands for which the scores would otherwise be ‘skewed’ due to the fact that only a few relevant pages had been identified).
Comments